8 Epilogue
In writing this book, I had three primary goals: to provide a comprehensive overview of complex-systems research with a particular emphasis on its applications in psychology and the social sciences, to teach skills for complex systems research, and to encourage critical thinking about the potential applications of complex systems in psychology. I will first discuss the main points of the chapters briefly and then focus on evaluating the complex-systems approach to psychology.
In Chapter 1 I defined complex systems. Complex systems are composed of interacting subsystems, resulting in emergent behaviors not seen at lower levels. These emergent patterns often arise through self-organization, can change suddenly, and may exhibit chaotic behavior, making prediction difficult. They can be studied using various methods, such as those originating in nonlinear dynamical system theory, agent-based modeling, and network theory.
I argued for the independence and autonomy of psychology as a scientific discipline. This does not mean that the mechanisms and principles that operate in psychological systems are necessarily different from those in other sciences. The synchronization of atoms in a laser beam may not be very different from the alignment of symptoms in a depressed patient or the synchronized movements of people evacuating a burning building. Complex systems in any science are almost always networks in which unpredictable phenomena can occur. These can be networks of atoms, neurons, symptoms, cars, people, or even entire countries. In these dynamic networks, there are usually only a limited number of organized patterns or stable states. Manipulating control variables can lead to bifurcations, which can take a limited number of forms. We have seen these principles again and again.
One may argue that emergent properties at one level of description can always be explained or rewritten as lower-level events, but this is largely irrelevant. In psychology we have to distinguish at least three basic levels of description related to the brain, the mind, and the social world. In describing the mind, delving deeper than the level of neurons does not provide additional insights.1 And in modeling the spread of conspiracy theories, the way axons of neurons grow can be left out of the equation.
I ended with reasons to be moderately optimistic about the application of complex-systems science in psychology. First, complex systems can often be simplified without losing their explanatory value. Second, these systems can be described by a limited number of equilibria. Third, we can use network science to model complex systems.
In Chapter 2, I introduced chaos theory and many of the key concepts of complex systems, such as fixed points, limit cycles, bifurcations, and dynamical system models. Learning about deterministic chaos changed my worldview and also my appreciation of beauty in mathematics. I hope I succeeded in conveying both.
It often seems to me that psychologists somehow believe that if they could collect vast amounts of extremely accurate life history, environmental, genetic, biological, and neuropsychological time-series data from millions of individuals—a feat that is currently unattainable—and feed it into a sophisticated nonlinear multilevel regression model with numerous higher-order interactions, they could predict virtually anything. This is simply wrong, not only because of deterministic chaos but also because of the influence of epigenetic processes (the third source), which I discussed in Section 4.3.4. The Pólya urn model provides a very simple demonstration of why even perfect knowledge of the initial conditions and dynamics of a system is insufficient to predict individual developmental outcomes.
The application of chaos theory in psychology is limited to the analysis of psychophysiological data. The hypothesis is that the brain works best on the edge of chaos (see also Section 5.4.1). Despite hundreds of papers written in the last forty years, I would say that the evidence for this hypothesis is inconclusive. This approach requires very high-quality data and advanced statistical approaches, which are difficult to acquire and challenging to develop. Furthermore, it is worth mentioning that there are even skeptics who question the applicability of chaos theory in studying complexity altogether (Anderson 1999).
Chapter 3 was devoted to transitions, or tipping points, as they are sometimes called. To me this is a very practical concept in complex-systems theory. I am probably somewhat biased, but I see instances of tipping points across a wide range of psychological processes. I list just a few that I have not discussed: sudden insights, creative breakthroughs, aggressive acts, the onset of puberty, mood swings, vocabulary spurts, and dropping out of school. In all these cases, the modeling and empirical program laid out in Chapter 3 might be fruitful. The chapter also contained an elementary introduction to the mathematics of bifurcations and catastrophes. I think a basic understanding of the key formal concepts is necessary and achievable. I provided sources for further study; for those who find these concepts still difficult, I also recommend running and studying the examples of the cusp catastrophe in Chapter 4. I have also outlined a methodology for empirically evaluating catastrophe models using catastrophe flags and Cobb’s statistical approach.
In Chapter 4, I focused on building dynamical systems models. I introduced Grind as a tool for implementing a wide range of dynamical systems models in biology and psychology. This modeling approach allows us to build more mechanistic models that we can still fully understand (using numerical bifurcation analysis). I have tried to give a representative overview of dynamical systems modeling in psychology, but you will easily find many other models in the literature. In such a case, I always recommend implementing the model yourself. In most cases, Grind will do. Replication is the key to good science, and you will learn a lot in the process.
I discussed the evaluation of ecosystem models in some detail (Section 4.2.7). I noted that even simple models imply a large number of assumptions, many of which are made implicitly (with the assumptions underlying the Lotka—Voltera model as an example). Also, seemingly trivial changes in model choices can have a huge impact on the qualitative behavior of models. As I said in the same chapter, I find the process of formalizing a verbal model fascinating. It tends to be very confusing. Suddenly it is unclear what the assumptions are, what mechanism is really being proposed, what the time scales are, or even what the phenomenon to be explained really is.
Models usually combine a number of mechanisms, and I strongly recommended reusing mathematical model pieces in other models. Modeling by analogy can be very productive (Haig 2005). Finally, when modeling, it is critical to always keep the connection to the data in mind. I am particularly concerned about this with the causal loop diagram approach. Models built in a session with content experts tend to get big with lots of boxes and links. This may not be a problem if all time series of measurements for each of these boxes are available, but this is rarely the case. I prefer to start simple and only add variables and equations when some established phenomena cannot be explained by the most trivial model.
In Chapters 2, 3, and 4, I focused on systems with a small number of variables. This part of the book covers what is often called nonlinear dynamical system theory. I view the theory of nonlinear dynamical systems as a fundamental component of the complex-systems approach. The second part of the book deals with systems with a large number of variables.
Self-organization was the subject of Chapter 5. I also used this chapter to introduce main theories in the study of complex systems, such as Haken’s work on synergetics and Prigogine’s ideas on irreversible transition and the second law of thermodynamics. I hope that I have successfully conveyed my own sense of awe and amazement at the self-organizing processes found in nature, as well as the unexpected potency of seemingly simple systems like the Game of Life. By engaging in the NetLogo simulations, I trust that the somewhat abstract concept of self-organization has become more comprehensible. As an example, I mention the spiral waves in the spatial model of hypercycles that prevented the abundant growth of parasites. This example of strong emergence is easy to understand by running the simulation and studying the basic NetLogo code.
I again realized while writing this book how the concepts of self-organizing complex systems were already present in early day psychology. I gave Gestalt psychology and Piagetian theory as examples, but one could make the same point for Rogers’s theory of self-concept, Heider’s balance theory, or Gibson’s ecological theory of perception. This is why I perceive the complex-systems approach in psychology less as a novel theory and more as a formalization of these intriguing yet abstract verbal theories. For instance, the Ising attitude model formalizes of numerous established concepts in social psychology.
Chapter 6 focused on the psychological and psychometric network approaches. This chapter ends with an extended discussion of the challenges facing this popular research line. My own contributions are mainly theoretical. I do think it is important to provide an alternative to the common cause view on psychological traits. The inability to intervene on common causes, or even to gain knowledge of these fixed biological factors without relying on the observable factors they explain, leads to a discouraging psychological theory that can easily be misused to abandon the less fortunate to their fate (Heckman 1995).
In terms of modeling, I consider the application to attitudes to be the most successful. The Ising attitude model not only builds on earlier connectionist network models, but also incorporates improvements. The dynamics of the Ising model are better understood from a mathematical perspective, it offers a novel psychological interpretation of the temperature parameter, and it can be effectively fit to data. The model formalizes key concepts in social psychology, such as dissonance and the mere thought effect, while suggesting a new explanation for the differences between implicit and explicit attitude measures. Using the mean-field approximation of this model in the HIOM represents an innovative development in sociophysics. I expect many more innovations in both network psychology and network psychometrics.
In the final chapter, Chapter 7, we moved into the realm of the social world. Because psychologists are often unfamiliar with disciplines such as computational social science, sociophysics, and agent-based modeling, I aimed to provide a concise overview of this rapidly evolving field. Finding the right balance in simplification has proven to be a challenge. While the simple voter model offers analytical tractability, its relevance is primarily theoretical. More realistic models quickly become intractable, even with the help of simulations.
I have focused on improving the psychological realism of the agents. My approach involves linking three levels of description: the interaction of attitude elements, the mean-field representation of attitudes as cusps, and the HIOM, where cusp-like agents interact within social networks. To my knowledge, this three-level integration is unique. Similar to many complex models in psychology and the social sciences, a notable weakness is the connection to data, specifically, data that really differentiate models.
Let’s zoom out more. By now, you should have a solid understanding of what complex systems involve, including the concepts of deterministic chaos, catastrophes, and self-organization. I have illustrated these ideas with numerous examples from various scientific fields, including psychology. It is vitally important to be aware of existing models and frameworks when you begin constructing your own models. This was my first goal.
Second, I placed significant emphasis on the importance of modeling skills. For me, the one critical path to comprehension lies in simulation. I hope that the skills you have developed by working through my exercises will encourage your engagement in formal modeling in psychology.
My third objective was to encourage a critical approach toward the study of complex systems in psychology and, in a larger context, within the realm of psychology and science itself. This naturally leads to the question of my personal stance on this undertaking. I must confess, I have mixed feelings.
About twenty years ago, in my inaugural lecture, I portrayed myself on the unstable maximum between the two minima of the cusp potential function. The attitude object was our field of psychology, and the minima represented positive (“love”) and negative (“hate”) evaluations. Since I am obviously highly involved in the matter, I’m on the front side of the cusp, which means that this in-between state is highly unstable, assuming, of course, that the cusp model of attitudes is correct.
So, if you are asking me to burn our field to the ground, including my own work, you have come to the right place. In short, we have a replication crisis (Aarts et al. 2015; Nosek et al. 2022; Pashler and Wagenmakers 2012), we have a theory crisis (Eronen and Bringmann 2021; Oberauer and Lewandowsky 2019), and we have a measurement crisis (Franz 2022; Lumsden 1976; Michell 1999). How many crises can you have?
Each of these crises is more severe than one might think at first glance. For a long time, questionable research practices, \(p\)-hacking, selective reporting, cherry-picking studies, presenting exploratory results as confirmatory results, to name a few, dominated research. All of this has changed radically since 2011. Surprisingly, a fraud case2 in my own country played a major role in this shift to open science, preregistration, and data sharing. Maybe we were in a metastable state and just needed one such perturbation. We are still in the midst of this transformation and should not be celebrating too soon (Chambers 2017). But a revolution it is!
Then the theory crisis. Depending on the criteria (weak or strong), psychologists either have millions of theories or none. There is not much in between. Many recent papers have proposed formalization as a way out of the theory crisis (Oberauer and Lewandowsky 2019; Borsboom et al. 2021; Rooij and Blokpoel 2020). This book is written from that perspective. I hope it makes a contribution, but I’m well aware of the differences between the scientific basis of formal models in the natural sciences and our modest attempts. To me, it is all about the right degree of simplification. In modeling complex systems, we almost always define at least two levels: the microscopic and the macroscopic. Emergent phenomena at the macroscopic level arise from microscopic interactions. The step from neural activity to higher reasoning just seems too large (although we may need to rethink this in light of the astonishing successes of large language models).
In writing this book, I learned that the distinction between phenomenological and mechanistic modeling is less discrete than I thought. First, we have been able to provide a foundation for some phenomenological catastrophe models. The cusp model of attitudes can be derived from the Ising model of attitudes, which is based on some simple assumptions about attitudinal networks. Second, many biological models combine phenomenological and mechanistic elements. Some terms may be well argued, others are just pragmatically chosen (the Holling types, for example). Nevertheless, I hope to have shown that studying these models is very informative. In my work, analogical modeling plays a central role.
I see the measurement crisis as the most serious problem. Let me recall Richard Feynman’s claim that the accuracy of calculating the size of the magnetic moment of the electron is the equivalent of measuring the distance from Los Angeles to New York, a distance of over 3,000 miles, to within the width of a human hair. And that was in 1985! I am an expert in psychological measurement and have published many papers on new methods of psychological measurement. I can tell you that we are nowhere near this amazing level of quantification and precision. We cannot do addition!
Addition is the litmus test of quantification (Michell 1997). Real quantities, such as weights and distances, can be added. One kilogram + one kilogram = two kilograms, which is twice as much as one kilogram.3 We cannot say such things about IQs, personality test scores, or Likert scores on attitude items. I do not think this is a hopeless endeavor. Even physicists have lived through times when key concepts were vaguely understood and poorly measured (see Inventing Temperature by Chang (2008)). I also draw hope from statements such as “Every law of physics, pushed to its extreme, will turn out to be statistical and approximate, not mathematically perfect and precise” (Wheeler 1994).
Currently, our scales of measurement are somewhere between ordinal and interval. Perhaps there is a continuous path to improvement. But for now, we have to live with rather weak scales of measurement. This has implications for our attempts to formalize psychological theories. The exact form of certain terms in our equations is irrelevant when we have only ordinal or semi-interval data. When testing models, we should focus on their qualitative behavior. This is exactly what we did with the catastrophe flags in Chapter 3, and it is one reason why I adhere to the sometimes-criticized catastrophe theory.
It is also important to temper expectations about distinguishing models based solely on their quantitative predictions. Achieving consensus on qualitative predictions may be the most feasible outcome. In the previous chapter, I used the example of segregation, which is predicted by many models and their variants, even when individuals are relatively tolerant.
I will not attempt to review these crises in depth, but I would like to suggest, in line with the book, that these crises form a mutualistic network (figure 8.1). Progress in resolving one crisis will have a positive impact on resolving others. If we can have more confidence in the empirical basis of many well-known psychological phenomena, theory development will benefit. Improved theories are necessary to advance measurement, and vice versa. In periods of rapid progress in sciences such as particle physics and biochemistry, we often see an upward spiral of theory development and measurement techniques.
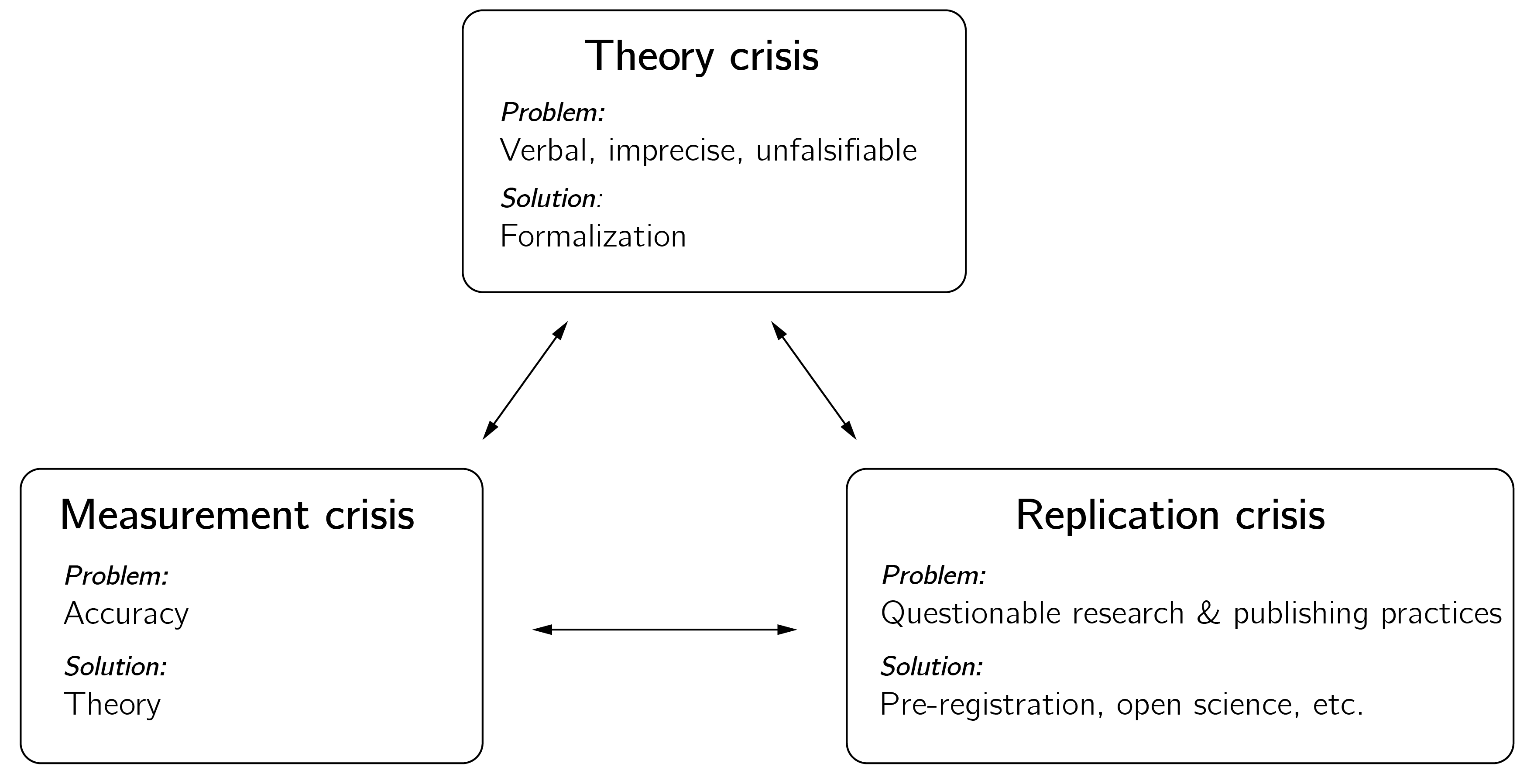
We have been part of at least one such radical transformation, and it is happening now: the AI revolution. Of course, we cannot claim ownership of this revolution, as many disciplines, such as computer science, have played a key role. But the current progress is based on mechanisms (neural learning and operant conditioning) that were first studied by psychologists. Unfortunately, there are no other convincing examples. We have not yet invented the plane or the refrigerator. This is no joke. In science, technology is the proof of the pudding. At some point we really must solve problems like addiction or panic disorder.
Despite all these negatives, psychology remains the most fascinating science of all. As I emphasized in Chapter 1, the emergence of global waves of electrical activity from rapid local interactions forms the basis of our conscious thought processes. This phenomenon is truly astonishing, and understanding it presents one of the most intriguing scientific tasks of all time. It involves the ultimate complex system, and we are exploring it with our own minds. Psychology is full of counterintuitive findings and paradoxes. Perhaps the greatest paradox is that psychology, unlike any other science, reveals the limitations and fallibilities of the human intellect while using the very intellect it studies.
The field of psychology is constantly moving away from grand theories, which were often little more than the collective opinions of some random man, toward more detailed models of subprocesses and systems. Significant progress has been made on a smaller scale. Similarly, the complex-systems approach does not currently provide a grand theory for psychology but, rather, a versatile set of tools for modeling, analysis, and understanding. I cannot conclude with a comprehensive overall theory of the complex human brain—mind—social world system. I simply don’t have it, only bits and pieces.
It is my hope that this book will contribute to lasting progress in psychological research. The overview of complex-systems research in other disciplines is perhaps helpful. I also hope that a new generation of researchers will learn many practical, useful modeling skills. And I hope that I have found the unstable maximum between hate and love for psychology.
Actually, the role of quantum processes in psychological processes is a topic of ongoing debate and research in the scientific community. While some researchers have proposed that quantum mechanics might play a role in cognitive processes, this idea is not widely accepted. One theory suggests that quantum processes in microtubules within brain cells could be linked to consciousness (Hameroff and Penrose 1996). Other researchers have suggested that the probabilistic nature of quantum mechanics might provide a better model for human decision-making than classical probability theory (Busemeyer and Bruza 2012). However, this work does not suggest that quantum physical processes are involved in decision-making. Rather, it uses quantum theory as a mathematical framework for modeling cognitive processes.↩︎
This is sufficient but not a necessary condition. Sometimes a concatenation of two quantities gives a weighted mean, for example when blending two liquids with varying temperatures.↩︎